AI's Impact On Biotechnology
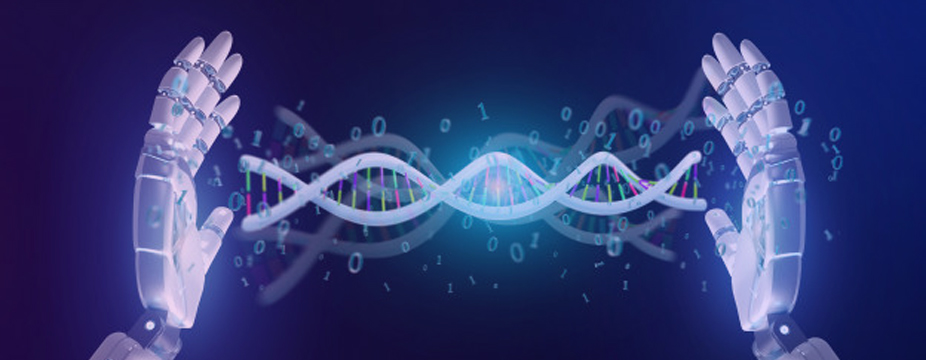
Biotechnology is a curious marriage of two seemingly disparate worlds. On one end, we have living organisms—wild, unpredictable celestial creations that can probably never be understood or appreciated enough, while on the other is technology—a cold, artificial entity that exists to bring convenience, structure and mathematical certainty in human lives. The contrast works well in combination, though, with biotechnology being an indispensable part of both healthcare and medicine. In addition to those two, there are several other applications in which biotechnology plays a central role—deep-sea exploration, protein synthesis, food quality regulation and preventing environmental degradation. The increasing involvement of AI in biotechnology is one of the main reasons for its growing scope of applications.
So, how exactly does AI impact biotechnology? For starters, AI fits in neatly with the dichotomous nature of biotechnology. After all, the technology contains a duality of its own—machine-like efficiency combined with the quaintly animalistic unpredictability in the way it works. In general terms, businesses and experts involved in biotechnology use AI to improve the quality of research and for improving compliance with regulatory standards.
More specifically, AI improves data capturing, analysis and pattern recognition in the following biotechnology-based applications:
Discovering and Monitoring New Marine Organisms
According to certain estimates, there are 15 million distinct species of living organisms on this planet. Out of those, only 2 million have been discovered yet. Additionally, about 80% of the world's rivers and oceans are unmapped and unexplored to date. Essentially, this means that freshwater and marine ecosystems may contain the largest number of unknown plant and animal species known to humans. Discovering and monitoring such organisms are consequential for industrial and environmental development, growth and maintenance. Such discoveries form the crux of marine biotechnology research. Newly discovered organisms are used in industries for the creation of new compounds and raw materials. Such materials then go on to become vital ingredients of finished goods such as brand-new medicines, foods and beauty products. Businesses involved in fishery also stand to benefit from the discovery of new types of fish, as they can sell them to boost their profits. From an environmental perspective, the monitoring will help to understand biodiversity in the oceans and take measures for the protection of rare species. As a result, involving AI in biodiversity for marine research helps to maintain ocean biodiversity.At the same time, deep-sea exploration is anything but easy, even for big and resourceful businesses. Explorers need to diligently spend thousands of hours underwater without a lot of maintenance support for the purpose. Even if they are covered in protective gear, with oxygen masks to support their breathing, they may suffer from cramps after spending that long in the extreme-pressure underwater surroundings. Additionally, oceans and seas contain highly saline and corrosive water that can cause damage to their protective gear, extraction machines and research equipment.
There are already several projects that feature AI algorithms developed and trained for marine discoveries. Microscopic organisms are useful for evaluating marine properties such as the temperature, acidity, salinity and nutrient concentration of specific regions. In such projects, leveraging AI and VR allows researchers to reconstruct ocean circulation and heat flow patterns accurately for understanding climatic patterns and reactions underwater.
Creating New Proteins
At some point, you’d have heard the saying, “proteins are the building blocks of life.” Although cliched, that line is true. As you probably know, proteins allow you to maintain a steady fluid balance and pH, aids your body's structural framework and build and repairs your cells and tissues. Additionally, there are several proteins that haven’t been created or discovered yet. The creation of proteins is a necessary task for healthcare, pharmaceutical and researchers and the development of consumer products.
One of the main challenges during artificial protein creation is the complexity attached to the process. Proteins are rarely, if ever, simple compounds with small molecular numbers. A simple organic cell contains holds about 42 million protein molecules. Creating proteins involves data analysis and pattern recognition of large amounts of generated information to gain insights. Once again, AI carries out the process as it can scan, absorb, clean, analyze data and provide output by scanning through a massive number of datasets used to train the algorithms used in these operations.
Adding to the complexity is the accurate analysis of the 3D chemical structure of proteins that determines their function. Traditionally, scientists use existing protein shapes and patterns for creating new ones. In the data-driven process, they alter the amino acids that a protein is composed of. Machine learning can use computational records to find physiochemical simulations to generate 3D models of new protein created on the basis of the amino acid sequence and the new protein.
An example of AI being used for protein creation is in drug development to mitigate diseases such as cancer. Researchers from the Chalmers University of Technology in Sweden have developed an algorithm-driven tool that can generate novel proteins. The researchers believed that the current model of introducing random mutations to protein sequences kept increasing the cost of the synthesis. Their tool, named ProteinGAN, uses generative deep learning to perform the task efficiently.
In the method, the researchers feed the AI algorithm millions of images and other types of data regarding well-studied proteins. Over a period of time, machine learning learns the common pattern commonalities in each protein. With such insights, new proteins can be created. After the creation, the tool also is trained to determine if synthetic proteins are real or fake by repeatedly comparing them to naturally occurring proteins until the point that it cannot differentiate between the two. As a result, the proteins created in a lab will retain the properties of their naturalistic counterparts.
The complex nature of proteins and their creation add to the cost of formulating them. ProteinGAN, and similar other models, enables protein developers to save a ton of costs by eliminating the workforce requirements in the process and greatly cutting down on time.
The next challenge in artificial protein synthesis is the use of AI in biotechnology to improve generated protein properties, such as reduced volatility, that will be useful for stable research operations with such proteins.
Creating Genetically Modified Foods
As you may know, meat consumption is one of the major contributors to environmental degradation through greenhouse gas generation. As a result, sustainability through consuming genetically modified foods instead of meat products is a growing concept today. In the US, a survey found that about 40% of Americans have tried plant-based meat foods created genetically in labs. An application similar to artificial protein creation is the production of such genetically modified foods. Like proteins, genetically modified foods, also known as GM foods, are complicated to develop and produce on a large scale with traditional methods.
The use of AI in biotechnology plays a prominent role in GM food creation as well. An American GM food producer named Yield10 uses its technological platform named GRAIN—an acronym for Gene Ranking Artificial Intelligence Network—to identify genomic targets for the improvement of crop yield and bioproduct contents. The system uses a plant's metabolism cycle to study how it can be modified. GRAIN runs through billions of public and privately available datasets before coming up with conclusive information regarding specifically identified genes. Essentially, the identified genes in certain plants can be modified to improve them. These GM plants and foods can be considered as alternatives to meat products, thereby reducing environmental degradation in an indirect way.
Post a Comment