Is Machine Learning or Deep Learning Best for Your AI Project?
Software developers face decisions on which advanced analytic techniques are within reason to incorporate. Viewing members of a team assembled to work on projects incorporating AI, the data scientist is likely to have the best grasp of the risks versus the rewards of different tools and approaches, suggests a recent article in Data Science Central.
Powerful and reasonably-mature machine learning techniques are the most widely adopted. Deep learning describes deep neural networks and reinforcement learning. Deep learning encompasses convolutional neural networks (CNNS), recurrent neural networks (RNNs), long short-term memory networks (LSTMs) and generative adversarial networks (GANs). In applications, these would cover image and video processing and search, text and audio processing, game play as optimization and several versions of time series forecasting.
However, deep learning solutions typically require a larger volume of data, are difficult to train and require specialized skills to build, implement and maintain. These all heighten the risk. So whether deep learning techniques should be recommended for a company’s digital journey needs to be carefully considered.
Both deep learning and machine learning techniques can address all classification, continuous estimating, clustering, optimization and anomaly detection functions.
A 2018 study from McKinsey Institute suggested deep learning techniques can boost the value derived from applications incorporating AI, by 30% to more than 100%. The travel, transport and logistics, retail, automotive assembly, high tech and oil and gas industries were seen as reaping the greatest advantage by incorporating AI into their digitization.
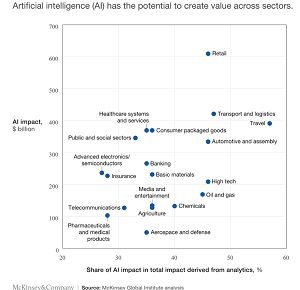
Author Bill Vorhies, President and Chief Data Scientist at Data-Magnum consultants, and a practicing data scientist since 2001, was not so sure McKinsey’s advice was the way to go. “ I’m not sure McKinsey has adequately assessed the difficulty, risks, or level of effort associated with such an enthusiastic embrace,” he wrote.
Maybe Data Science Without Machine Learning is Enough
Sometimes data science all by itself without even incorporating machine learning is the way to go, suggests a recent account in The Enterprisers Project. “At the end of the day, most of the algorithms use statistical techniques,” stated Anil Vijayan, Vice President at Everest Group. “Not every problem requires AI to solve though. In many cases, using “traditional” data science may not just suffice, but also be more efficient.”
Another way to look at it is to consider deep learning models as a “rocket engine” whose fuel is the huge amount of data fed into its algorithms, suggests a recent article in edureka. Deep learning is a special type of machine learning that was inspired by the functionality of brain cells in what is called an artificial neural network. It adjusts data connections between artificial neurons according to the data pattern. More neurons are needed if the data volume is large. The model learns automatically at multiple levels of abstraction, allowing complex functions to be delivered without depending on a specific algorithm.
In a project to learn to identify the difference between a cat and a dog, a deep learning model can automatically discover which features are important for classification, whereas in machine learning, the developer or data scientist needs to manually provide the features. In conclusion, deep learning is seen as the next evolution of machine learning.
Have Another Wrinkle, the Third Wave of AI
Just when you thought you had this all figured out, along comes the “third wave of AI,” in which AI systems not only learn and reason as they encounter new tasks, but they have the ability to explain their decision-making, suggests Tolga Kurtoglu, Head of Global Research, Xerox PARC, in a recent article published at techradar.com.
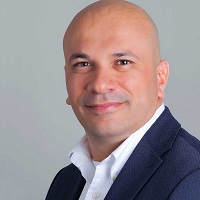
We are in the second wave of AI right now, with systems with nuanced classification and prediction capabilities, but no contextual capability and minimal ability to reason. Major machine learning AI platforms including IBM’s Watson and Salesforce’s Einstein are able to synthesize large volumes of data to provide insight and answers, but they are not able to fully explain how they got the answer.
Most AI systems today are based on machine learning, which requires thousands, if not millions, of data examples to work property. To enable widespread adoption in the third wave of AI, AI systems need to shift away from this data-heavy approach, the author suggests.
He envisions a combination of approaches being used, including systems modeling and human-machine collaboration. The hybrid approach will allow organizations to experience the benefits of AI with a fraction of the data required for large machine learning platforms. And it will help solve problems for which large datasets do not exist.
The third wave of AI, he suggests, has the promise of having humans perceive AI as a trusted partner in addressing complex challenges.
Read the source articles and see references at Data Science Central, McKinsey Institute, The Enterprisers Project, edureka and at techradar.com.
Post a Comment